Research Article, J Appl Bioinforma Comput Biol Vol: 11 Issue: 5
Mutation Based Network Analysis to Predict the Affected Pathways for Sars Cov-2 Variants Isolated from the Kingdom of Bahrain
Khalid M Bindayna1*, Khaled Saeed Tabbara1, Kassim Aradati2, Ronni Mol Joji1 and Haitham Jahrami1
1Department of Microbiology, Immunology and Infectious Diseases, Arabian Gulf University, Manama, Kingdom of Bahrain
2Department of Medical Sciences, Bahrain Specialist Hospital, Manama, Kingdom of Bahrain
*Corresponding Author: Khalid M Bindayna, Department of Microbiology, Immunology and Infectious Diseases, Arabian Gulf University, Manama, Kingdom of Bahrain, E-mail: bindayna@agu.edu.bh
Received date: 27 April, 2022, Manuscript No. JABCB-22-61940;
Editor assigned date: 29 April, 2022, PreQC No. JABCB-22-61940 (PQ);
Reviewed date: 13 May, 2022, QC No. JABCB-22-61940;
Revised date: 20 May, 2022, Manuscript No. JABCB-22-61940 (R);
Published date: 27 May, 2022, DOI: 10.4172/2329-9533.1000232
Citation: Bindayna KM, Tabbara KS, Aradati K, Joji RM, Jahrami H (2022) Mutation Based Network Analysis to Predict the Affected Pathways for Sars Cov-2 Variants Isolated from the Kingdom of Bahrain. J Appl Bioinforma Comput Biol 11:5.
Abstract
SARS-associated coronavirus (SARS-CoV) causes respiratory illness called severe acute respiratory syndrome (SARS). We have identified the SARS-COV2 variants from the kingdom of Bahrain. In this study, we applied network theory to predict the effect of a mutation in the SARS-COV2 protein interaction network with the human interactome. For the lineage analysis, first, we have downloaded the FASTA file of 874 variants identified in the Kingdom of Bahrain by comparing with hCoV-19/Wuhan/WIV04/2019 strain (isolated from Wuhan, China) as a reference for variant. Our network analysis predicts the drug target by constructing a protein-protein interaction network of SARS-COV2 with the human interactome and eventually get 36 mutations on spike protein. We have a total of 929 unique interactions among the human proteins that are interacting with SARS-COV2 proteins. This gives us the actual view of how SARS-COV2 interacts with human interactome. We find out the point mutations in the available SARS-COV2 variants which are still a potential threat for the vaccinated people. Nine SARS-COV2 proteins that are reported in the SARS-COV2 protein-protein interaction network. The variants are associated with a mutation in S, NSP12, N, N, NSP6, NSP1, NSP15, NSP13, NSP2, NSP5 and NSP8. We have selected only those proteins which undergo a change inbetweenness centrality >=0.01. The effect of these variant’s on the human interactome are associated to affect different regulatory pathways of the cell. A number of research laboratories are making a major effort to develop therapeutic strategies for controlling COVID-19. These variants can be a potential therapeutic target to develop a drug that will prevent viral entry into target cells and virus replication.
Keywords: SARS-associated coronavirus (SARS-CoV); Network theory; SARS-COV2 protein-protein interaction network; Bahrain; Therapeutic target
Introduction
Severe acute respiratory syndrome coronavirus 2 (SARS-CoV-2) is caused by the SARS-CoV-2 virus that needs fast and effective drugs to save peoples’ lives. The global impact of the SARS-CoV-2 requires different ways for finding treatments and vaccines to reduce the rate of morbidity and mortality throughout the world. In this COVID-19 context, finding targets for drug repurposing can be an effective way to present new fast treatments. Recent studies predict new drug targets or drug repurposing candidates by using computational methods. Identifying drug targets that are approved and estimating their therapeutic potential for COVID-19 [1]. Scientific study reveals that SARS-CoV-2 infects human cells by hijacking the host's translation mechanism to produce 29 viral proteins. These proteins interact with human proteins to start the other viral processes needed for viral duplication. It has been identified that 332 human proteins are involved in the binding of these viral proteins. Out of these 332 proteins, 67 human proteins as drug targets were identified . The identification of host proteins and viral protein reliance during infection can provide significant insights into finding suitable drug targets for developing antivirals medicine against SARS-CoV-2 [2]. Analysis of a large amount of data in the biological process helped us for a better understanding of cellular mechanisms. This kind of data is usually represented in the form of a network. One of the most important biological networks constructed from experimental data is the Protein-Protein Interaction (PPI) network [3].
Between 1 January and 31 May 2021, 665 people died from COVID-19 out of this 90% were unvaccinated. On June 1, the number of critical COVID-19 patients was 326, and 83% were unvaccinated. The Ministry of Health announced raising the country's daily vaccination capabilities to 31,000 patients per day. On 3 June 2021, the country's National Health Regulatory Authority approved Sotrovimab, which is a monoclonal antibody used for emergency use against COVID-19 patients. In the same month, Bahrain's sovereign wealth fund mumtalakat signed a memorandum with the Russian Direct Investment Fund and Binnopharm to manufacture and export the Sputnik V vaccine in the MENA region to prevent the further progression of conditions.
In June 2021, it was reported that Bahrain is planning a booster shot of the Pfizer vaccine for the elderly and health-compromised individuals who already received BBIBP-CorV. Due to the efficacy of the Chinese vaccine to protect those populations from covid illness, the nation was able to reduce infections 8-fold from May 29 to June 23, 2021 by using the sinopharm vaccine. The COVID-19 vaccines are widely credited for their role in reducing the spread, severity, and deaths caused by COVID-19. This knowledge accelerated the development of various vaccine preliminaries directed to prevent symptomatic and often severe illness due to covid 19.
Unlike other viruses, the rates of mutations in the novel coronavirus, which causes COVID-19 infection, is relatively very low, which is about half of the rate of influenza (flu) virus and a quarter of the HIV rates. Mutations produce variability within a population, which allows natural selection to modify traits that are beneficial or detrimental to a cell. The coronavirus currently has 12,700 mutations that have been identified, there are 12 main types of the virus (identified as 19 A, the original type, through 20 J), also five strains and almost more than 4000 variants. The strains are known as L, which mutated into the S strain followed by V and G (further mutating to GR, GV and GH and several infrequent mutations collectively grouped as O). The G strains are now the dominant strain around the world. SARS-CoV-2 variants with a spike (S)-protein D614G mutations have become the most common variant. It is so named because one amino acid is changed from a D (aspartate) to a G (glycine) at position number 614 of the viral spike proteins. The spike protein mediates the binding to the target receptors and the fusion to the human cell membrane [4]. The S protein extends from the viral membrane giving the virus surface a crown-like appearance, for which the virus is named; corona is crown in Latin. Most of the variants of concern contain mutations in the Receptor-Binding Domain (RBD). It seems that these mutations are responsible for increased virulence and immune evasion potency. It is known that the RBD is involved in viral recognition and cell receptor binding and interaction, thus any structural changes seem to be directly related to viral transmissibility and virulence. It has also been identified in numerous studies that antibodies developed against the RBD have been found to have maximum potency against the SARS-CoV-2 [5].
Our analysis could lead to the identification of new drug targets for the variants isolated from the Kingdom of Bahrain. We eventually end up with 36 mutations on spike protein. In this work, Our network analysis predicts the drug target from all the available SARS-COV2 genomes isolated from the Kingdom of Bahrain in terms of their variants and mutations. We have constructed a protein-protein interaction network of SARS-COV2 with the human interactome. Based on the topological analysis of the variant proteins, we have predicted protein targets that could hamper the reaction cascade of SARS-COV2 proliferation inside a human cell. Based on the point mutations, we have pointed out significant proteins that have been modified in these variants.
Results
Lineage and mutant determination
We have analyzed the various SARS-COV2 isolates of Bahrain and categorized them according to their lineage and type of variants. For the lineage analysis, first, we have downloaded the FASTA file of 874 variants identified in the Kingdom of Bahrain. With the help of the FASTA files, we have analyzed their lineages in the pangolin database [6]. The lineage distribution of the SARS-COV2 variants is described in Figure 1.
Variant identification
We have fetched a total 874 genomes of SARS-COV2 from the Global Initiative on Sharing All Influenza Data servers which are isolated from the Kingdom of Bahrain [7]. Multiple sequence alignment was performed for the detection of SNPs. We have used the Multiple Sequence Comparison by Log-Expectation algorithms to align the sequences [8]. We have used hCoV-19/Wuhan/WIV04/2019 strain (isolated from Wuhan, China) as a reference for variant and mutation analysis of 874 SARS-COV2 genomes isolated from the Kingdom of Bahrain. We have used the CoV server mutant analysis tool from the GISAID server. We have categorized the identified mutants into two categories;
• The variants which are reported previously
• The variants are unique to the Kingdom of Bahrain. All the variants are summarized in Table 1. Here we have only restricted ourselves with only the top 1% mutations based on their frequencies.
Existing mutation list | ||
---|---|---|
Mutation | Frequencies | Percentage |
spike_d614g | 177 | 7.898259705 |
nsp12_p323l | 133 | 5.934850513 |
nsp3_a994d | 72 | 3.212851406 |
ns3_q57h | 49 | 2.186523873 |
nsp3_t73i | 36 | 1.606425703 |
nsp3_q1884h | 35 | 1.561802767 |
nsp6_l37f | 35 | 1.561802767 |
nsp1_e37d | 24 | 1.070950469 |
spike_l452r | 24 | 1.070950469 |
spike_p681r | 24 | 1.070950469 |
n_d377y | 23 | 1.026327532 |
ns3_s26l | 23 | 1.026327532 |
ns7a_v82a | 23 | 1.026327532 |
Unique mutation list | ||
Mutation | Frequencies | Percentage |
nsp15_s243i | 12 | 9.090909091 |
nsp3_f1503i | 12 | 9.090909091 |
nsp12_s115a | 10 | 7.575757576 |
ns3_v225l | 6 | 4.545454545 |
n_e323k | 5 | 3.787878788 |
nsp13_a321s | 5 | 3.787878788 |
nsp2_c177s | 5 | 3.787878788 |
nsp5_e47d | 3 | 2.272727273 |
spike_r683l | 3 | 2.272727273 |
m_e11d | 2 | 1.515151515 |
nsp16_h119q | 2 | 1.515151515 |
nsp16_n196s | 2 | 1.515151515 |
nsp2_n282s | 2 | 1.515151515 |
nsp3_a274s | 2 | 1.515151515 |
nsp3_a99v | 2 | 1.515151515 |
nsp3_r30m | 2 | 1.515151515 |
nsp5_k236n | 2 | 1.515151515 |
nsp8_d163n | 2 | 1.515151515 |
spike_v1164a | 2 | 1.515151515 |
Table 1: Existing and unique variant list identified from the Kingdom of Bahrain.
Network analysis
We obtained 482 interactions of SARS-Cov2 proteins with the human interactome from the previous literature Gordon et al. In order to build the whole protein-protein interaction network, we have identified the interactions among the human proteins that are interacting with SARS-COV2 proteins, from the STRING database. We have total of 929 unique interactions. The whole PPIN consist of 482 interactions among SARS-COV2 proteins with human interactome and 929 other interactions among the human proteins that are interacting with SARS-COV2 proteins. Now the combination of these two subsets gives us the actual view of how SARS-COV2 interacts with human interactome. The PPIN is represented in Figure 2.
Basic network characteristics
The degree distribution of the network seems to be scale-free with an average degree of 7.04. Although we have connected the human proteins that are interacting with the SARS-COV2 proteins, this scalefree nature indicates that there are few proteins that are highly connected [9]. Figure 3 indicates the scale-free nature of degree distribution of the SARS-Cov2 proteins with human interactome.
Other than degree we have also explored the betweenness centrality, closeness centrality, clustering coefficient and eccentricity as shown in Figure 4.
The average degree of the network is 7.04 but as it is a scale-free network the diversity of degrees is very high. Though 7.04 for a network of 1411 nodes seems to be dense.
Network-based analysis of the variants
Now we have the protein-protein interaction network of SARSCOV2 proteins with human interactome. We also have the variant list identified from Bahrain. The next question we had was what are the implications of these variants on the proteins which will form the protein interaction network inside the human body. We have analyzed the effect of each of the variants on the whole PPIN network of the SARS-COV2 and human interactome [10].
In order to determine the effect of a particular point mutation (for a variant) in other interactions in the protein interaction network, we use betweenness centrality based differential network analysis. The betweenness centrality of a node V is given by the expression:
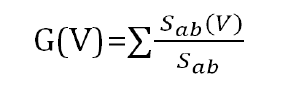
Where Sab is the total number of shortest paths from node a to node b and Sab (V) is the number of those paths that pass through V. If we take a differential betweenness value of each node for each variant point mutation (node deletion), we could observe the other proteins that are affected (change in betweenness centrality value >=1) highly.
In table 1 we found 9 SARS-COV2 proteins that are reported in the SARS-COV2 protein-protein interaction network. The variants are associated with a mutation in S, NSP12, N, N, NSP6, NSP1, NSP15, NSP13, NSP2, NSP5 and NSP8.
We have calculated the Z score of the betweenness change of each human protein after the point mutation i.e. node deletion. We have selected only the proteins which undergo a change in-betweenness centrality >=0.01. The list of affected proteins due to the variant is listed in Table 2.
S variants | NSP12 variants | NSP13 variants | NSP8 variants | ||||
---|---|---|---|---|---|---|---|
GOLGA7 | 0.2101 | AKAP8 | 0.6355 | orf9b | 0.51026 | DDX10 | 0.40157 |
ZDHHC5 | 0.2011 | TYSND1 | 0.6016 | M | 0.50768 | LARP7 | 0.28078 |
TUBGCP2 | 0.1094 | TRIM59 | 0.5259 | RAB8A | 0.40284 | RPL36 | 0.27856 |
EXOSC5 | 0.101 | UBAP2L | 0.4805 | RAB7A | 0.38741 | RIPK1 | 0.2768 |
MYCBP2 | 0.3834 | DCTPP1 | 0.3794 | nsp12 | 0.19982 | ||
N variants | LARP4B | 0.3261 | nsp12 | 0.3149 | TBK1 | 0.19944 | |
CSNK2A2 | 0.1049 | UBAP2 | 0.3148 | FASTKD5 | 0.29754 | RRP9 | 0.17936 |
ZYG11B | 0.2576 | TBK1 | 0.29095 | CDK5RAP2 | 0.17725 | ||
NSP6 variants | ECSIT | 0.2562 | USP54 | 0.26961 | SEPSECS | 0.16495 | |
SIGMAR1 | 0.4138 | RIPK1 | 0.2367 | SLC25A21 | 0.25604 | SRP72 | 0.15655 |
EXOSC3 | 0.1392 | PDZD11 | 0.2341 | nsp7 | 0.22164 | EXOSC8 | 0.14574 |
USP54 | 0.2306 | PRKACA | 0.21014 | SIL1 | 0.11671 | ||
NSP1 variants | MEPCE | 0.2214 | RDX | 0.20179 | MRPS5 | 0.11523 | |
PRIM1 | 0.19267 | ITGB1 | 0.2136 | orf8 | 0.18602 | PABPC1 | 0.11407 |
POLA1 | 0.17456 | DDX10 | 0.1753 | CSDE1 | 0.17811 | SRP19 | 0.10548 |
COLGALT1 | 0.15365 | AKAP9 | 0.1663 | HSBP1 | 0.17492 | PMPCA | 0.10414 |
MARK3 | 0.14125 | orf8 | 0.1649 | TOMM70A | 0.17229 | SRP54 | 0.104 |
AKAP9 | 0.10751 | nsp8 | 0.1566 | PRKAR2A | 0.17215 | ||
FAR2 | 0.1541 | CIT | 0.17197 | ||||
NSP15 variants | RAP1GDS1 | 0.1396 | PRKAR2B | 0.17178 | |||
ARF6 | 0.2395 | PPIL3 | 0.1327 | PCNT | 0.1575 | ||
NUTF2 | 0.2137 | nsp13 | 0.1314 | PDE4DIP | 0.15742 | ||
AKAP8L | 0.1297 | USP13 | 0.15205 | ||||
NSP2 Variants | EIF4H | 0.1291 | STOML2 | 0.14873 | |||
FKBP15 | 0.31021 | orf3a | 0.1277 | GORASP1 | 0.14524 | ||
RAP1GDS1 | 0.29430 | RBX1 | 0.1191 | C1orf50 | 0.12967 | ||
SLC27A2 | 0.20433 | nsp2 | 0.1176 | ATP6V1A | 0.12771 | ||
POR | 0.15593 | CWC27 | 0.1081 | GOLGA2 | 0.12686 | ||
GIGYF2 | 0.14923 | AGPS | 0.1081 | GRIPAP1 | 0.12446 | ||
EIF4E2 | 0.12235 | CSDE1 | 0.1071 | ||||
CDK5RAP2 | 0.1066 | ||||||
NSP5 variants | |||||||
HDAC2 | 0.41215 |
Table 2: Affected proteins with their Z score change in betweenness centrality value in human interactome which is affected due to variants point mutation.
Pathway enrichment for variants
In order to understand the effect of these variants in human cells we have enriched the GO pathway analysis using the Reactome server to check the possible alterations in the pathophysiology of the variant.
Effect of S variant
The effects of s variant on human interactome are primarily associated with transcriptional shutdown due to mRNA destabilization and ER stress (Table 3).
Name | pValue | FDR |
---|---|---|
Tristetraprolin (TTP, ZFP36) binds and destabilizes mRNA | 0.00531 | 0.05132 |
Butyrate Response Factor 1 (BRF1) binds and destabilizes mRNA | 0.00531 | 0.05132 |
KSRP (KHSRP) binds and destabilizes mRNA | 0.00559 | 0.05132 |
mRNA decay by 3' to 5' exoribonuclease | 0.00671 | 0.05132 |
ATF4 activates genes in response to endoplasmic reticulum stress | 0.0095 | 0.05132 |
PERK regulates gene expression | 0.01172 | 0.05132 |
RAS processing | 0.01283 | 0.05132 |
Table 3: Effect of s variant on human interactome.
Effect of NSP12 variant
The effects of the NSP12 variant on the human interactome are primarily associated with the cleaving of proximal proteins and their import. On the other hand, the N variant effect on CSNK2A2 protein which is associated with WNT mediated activation of DVL (Table 4).
Name | pValue | FDR |
---|---|---|
TYSND1 cleaves peroxisomal proteins | 0.00016 | 0.041 |
Potential therapeutics for SARS | 0.00123 | 0.12971 |
Wax and plasmalogen biosynthesis | 0.00156 | 0.12971 |
Localization of the PINCH-ILK-PARVIN complex to focal adhesions | 0.00921 | 0.17701 |
Peroxisomal protein import | 0.01057 | 0.17701 |
Defective RIPK1-mediated regulated necrosis | 0.0115 | 0.17701 |
MET interacts with TNS proteins | 0.0115 | 0.17701 |
Table 4: Effect of NSP12 variant on human interactome.
Effect of NSP6 variant
The effects of the NSP6 variant on the human interactome is also primarily associated with transcriptional shutdown due to mRNA destabilization (Table 5).
Name | pValue | FDR |
---|---|---|
Tristetraprolin (TTP, ZFP36) binds and destabilizes mRNA | 0.00266 | 0.01344 |
Butyrate Response Factor 1 (BRF1) binds and destabilizes mRNA | 0.00266 | 0.01344 |
KSRP (KHSRP) binds and destabilizes mRNA | 0.0028 | 0.01344 |
Table 5: Effect of NSP6 variant on human interactome.
Effect of NSP1 variant
The effect of the NSP1 variant on the human interactome is primarily associated with DNA damage and cell cycle regulation (Table 6). Whereas the NSP15 variant affects MET receptor recycling.
Name | pValue | FDR |
---|---|---|
Inhibition of replication initiation of damaged DNA by RB1/E2F1 | 0.000017 | 0.00013 |
Polymerase switching | 0.000019 | 0.00013 |
Leading Strand Synthesis | 0.000019 | 0.00013 |
DNA replication initiation | 0.000019 | 0.00013 |
G1/S Transition | 0.000023 | 0.00013 |
Table 6: Effect of NSP1 variant on human interactome.
Effect of NSP13 variant
The effects of the NSP13 variant on the human interactome are primarily associated with cell cycle regulation and the CaM pathway as shown in Table 7.
Name | pValue | FDR |
---|---|---|
ROBO receptors bind AKAP5 | 0.0000001 | 0.0000347 |
M Phase | 0.0000003 | 0.0000347 |
CREB1 phosphorylation through the activation of Adenylate Cyclase | 0.0000006 | 0.0000347 |
Calmodulin induced events | 0.0000006 | 0.0000347 |
CaM pathway | 0.0000006 | 0.0000347 |
Ca-dependent events | 0.0000011 | 0.0000501 |
Regulation of PLK1 Activity at G2/M Transition | 0.0000013 | 0.0000501 |
Table 7: Effect of NSP13 variant on human interactome.
Effect of NSP2 variant
The effect of the NSP2 variant on the human interactome is primarily associated with bile acid synthesis and regulation as shown in Table 8. On the other hand, the NSP5 variant affects HDAC2 which in turn affect cell cycle regulation via SC1.
Name | pValue | FDR |
---|---|---|
Synthesis of bile acids and bile salts via 24-hydroxycholesterol | 0.0002066 | 0.0057853 |
Synthesis of bile acids and bile salts via 7alpha-hydroxycholesterol | 0.000616 | 0.008624 |
Synthesis of bile acids and bile salts | 0.0012823 | 0.0109529 |
Bile acid and bile salt metabolism | 0.0015647 | 0.0109529 |
Table 8: Effect of NSP2 variant on human interactome.
Effect of NSP8 variant
The effects of the NSP8 variant on the human interactome are primarily associated with translational regulation as shown in Table 9.
Name | pValue | FDR |
---|---|---|
Translation | 0.0000026 | 0.0003702 |
SRP-dependent cotranslational protein targeting to membrane | 0.0000135 | 0.0009566 |
ZBP1(DAI) mediated induction of type I IFNs | 0.0003906 | 0.01836 |
Major pathway of rRNA processing in the nucleolus and cytosol | 0.001633 | 0.0498554 |
rRNA processing in the nucleus and cytosol | 0.0021152 | 0.0498554 |
Table 9: Effect of NSP8 variant on human interactome.
Discussion
SARS-CoV-2 is responsible for the pandemic of respiratory tract infection ongoing worldwide. Some of the patients are asymptomatic, showing mild symptoms, sometimes showing complications like pneumonia and acute respiratory distress syndrome (ARDS) etc. Systemic complications like cardiovascular disorders, persistent lung injuries and fibrosis are the key threats in addition to respiratory syndrome. Though the possibility of spreading the virus is slow, it is assumed that the infection will remain entrenched in the population for years. At that time though we have some approved vaccines available, but the absence of some therapeutic drugs is the main obstacle to controlling the death due to infection of coronavirus. As the development of drugs required detailed research and testing, we need some parallel approaches to hypothesize and test a probable drug. With the help of a rigorous computational approach, we find out the point mutations in the available SARS-COV2 variants which are still a potential threat for the vaccinated people. In this work, we applied network theory to predict the effect of a mutation in the SARS-COV2 protein interaction network with the human interactome. The identified proteins in the human interactome were enriched to predict the affected pathways in the human system. The proteins which are affected due to the point mutation in the variants could be the potential drug targets. It turns out that most of the SARS-COV2 virus mutations are associated with the translational shutdown and cell cycle block whereas some of them are associated with bile acid regulation etc.
The identified pathways could be the crucial pathways that are required for maintaining SARS -COV 2 infection and replication inside the cell. The identified proteins could be the potential agonist and antagonist of various replication machinery that the SARS-COV 2 virus used. The uniqueness of our research is that it not only explored the only interacting proteins of human interactome with SARS-COV2 interactome, but we also have considered the connecting links between the human proteins which could be the potential links to influence a cellular process. Our method is more generalized and could be more accurate to find out drug targets and affected pathways [11].
We mainly focused on the variants identified from the Kingdom of Bahrain however this powerful approach could lead to uncovering various mutational consequences and drug target problems of SARSCOV2 challenges.
Author Summary
The global pandemic of SARS-associated coronavirus (SARSCoV2), is a most critical challenge for researchers, to develop both therapeutic and immunogenic approaches against the virus. Major effort’s are made by a number of research laboratories to develop a therapeutic strategies for controlling SARS-CoV2 infection. In this context, we perform protein to protein interaction of human interactome with SARS-CoV2 proteins. We found a total of 929 unique interactions among the human proteins that are interacting with SARS-COV2 proteins. Apart from this we found point mutations in the available variants in SARS-CoV2 major protein (S, NSP12, N, N, NSP6, NSP1, NSP15, NSP13, NSP2, NSP5 and NSP8). These variants can be a drug target in the future research.
Acknowledgments
All the author’s contributed equally in research work and paper writing as well.
References
- Habibi M, Taheri G, Aghdam R (2021) A SARS-CoV-2 (COVID-19) biological network to find targets for drug repurposing. Sci Rep 11: 1-15.
- Habibi M, Taheri G (2021) Topological network based drug repurposing for coronavirus 2019. PloS one 16: e0255270.
- Bindayna KM, Deifalla AHAFS, Mokbel HEM (2021) Identification of E484K and other novel SARS-COV-2 variants from the Kingdom of Bahrain. Microb Pathog 157: 104955.
- Lauring AS, Hodcroft EB (2021) Genetic variants of SARS-CoV-2—what do they mean? Jama 325: 529-31.
- Tang JW, Tambyah PA, Hui DSC (2021) Emergence of a new SARS-CoV-2 variant in the UK. J Infect 82: e27-e8.
- O’Toole Á, Scher E, Underwood A, Jackson B, Hill V, et al. (2021) Assignment of epidemiological lineages in an emerging pandemic using the pangolin tool. Virus Evol 7: veab064.
- Shu Y, McCauley J (2017) GISAID: Global initiative on sharing all influenza data–from vision to reality. Euro Surveill 22: 30494.
- Edgar RC (2004) MUSCLE: multiple sequence alignment with high accuracy and high throughput. Nucleic Acids Res 32: 1792-7.
- Tripathi S, Kessler DA, Levine H (2020) Biological networks regulating cell fate choice are minimally frustrated. Phys Rev Lett 125: 088101.
- Lee W-P, Tzou W-S (2009) Computational methods for discovering gene networks from expression data. Brief Bioinform 10: 408-23.
- Feng Y, Wang Q, Wang T (2017) Drug target protein-protein interaction networks: a systematic perspective. Biomed Res Int 2017: 1289259.